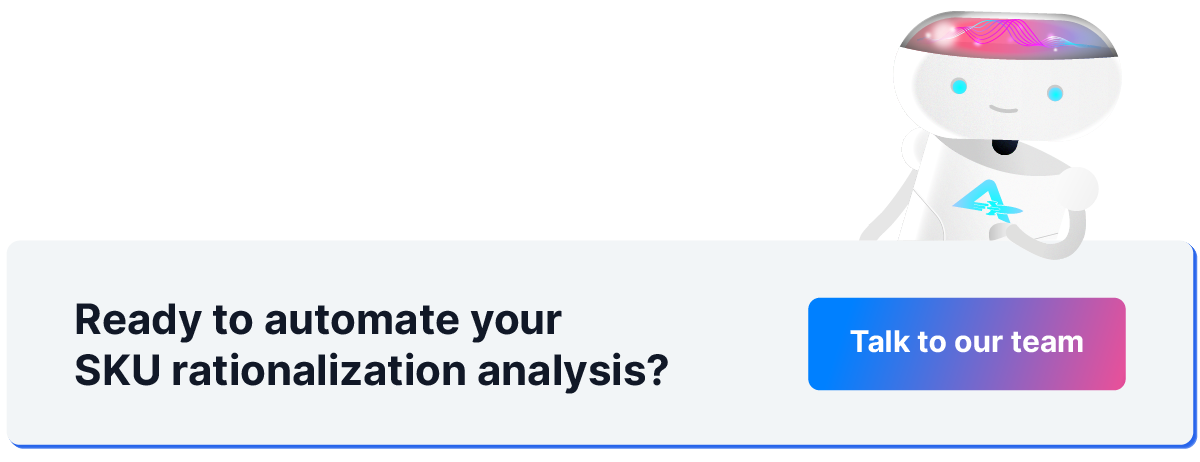
Throughout last year, the COVID-19 pandemic severely affected businesses. It impacted their ability to make accurate predictions, fulfill consumer expectations, and assess performance.
Restaurants were closed or shut down permanently. The financial sector adapted to a decrease in business profits and consumer disposable income.
Some CPGs and retailers coped with out-of-stocks and delayed supply chains, while others capitalized on “free” product trials as consumers flocked to whatever items were available during periods of pantry stocking.
Thus, many companies are poised for portfolio evaluations, whether to tighten their operational costs or to gain more market share.
Portfolio health can be enhanced by reducing products, changing factors like price, and investing in winners. To make these decisions, businesses require an examination of individual SKU performance, which is far easier said than done.
SKUs can be affected by many factors, such as segment, geography, price, promotions, channel, retailer, distribution, or competitors–and all to varying degrees.
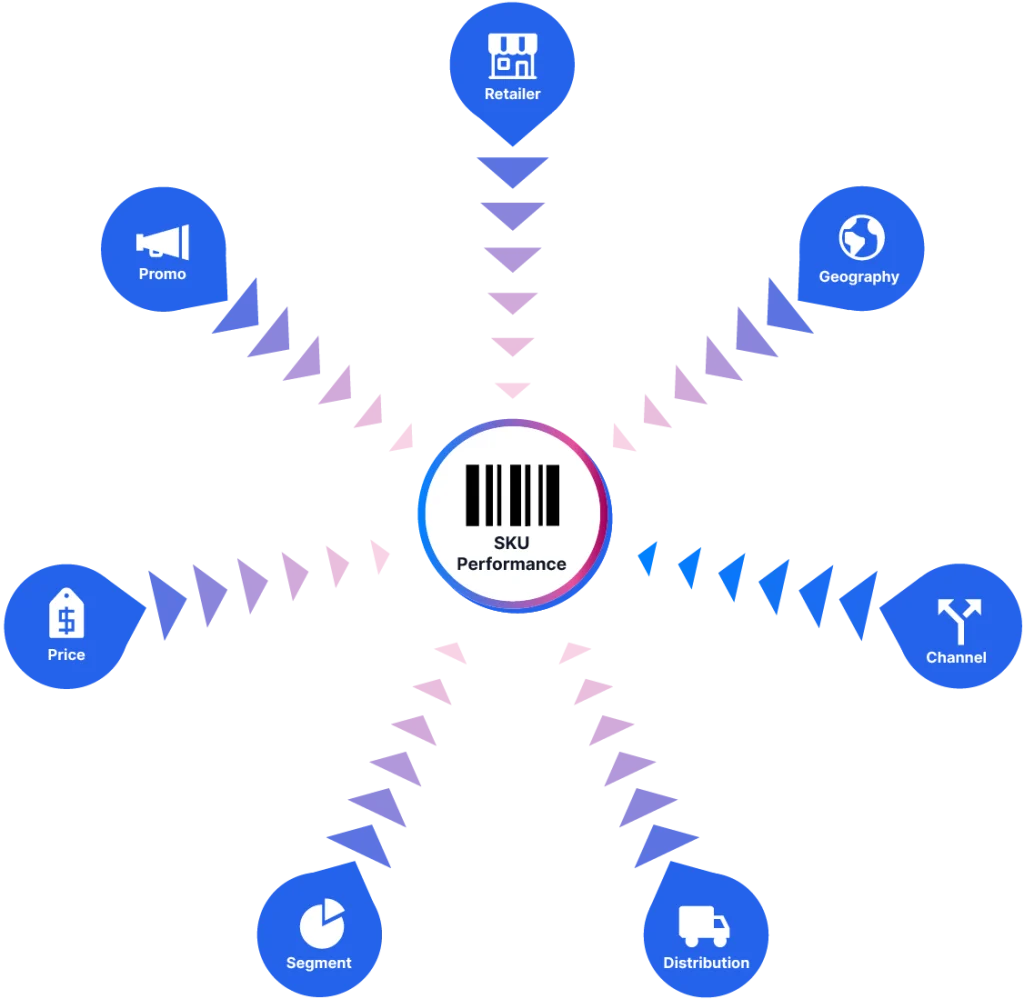
Analysts must grapple with these factors to gain an accurate view of SKU performance, but most businesses still struggle to determine their big-picture portfolio performance.
SKU rationalization, and how you approach it, can make a difference.
What is SKU Rationalization and Why Does it Matter?
SKU rationalization is the process of determining which products should be kept, retired, or improved based on the myriad of factors that contribute to performance. Sometimes referred to as SKU optimization, this process enables organizations to refine their product portfolios to improve their financial outlook.
By prioritizing SKUs that drive growth and cutting the tail on laggards, leaders can build healthier portfolios of revenue-generating, valuable products.
In addition to choosing which SKUs to keep and cut, analysts might also focus on solving product cannibalization to ensure SKUs aren’t competing with each other.
By understanding performance at the SKU level, companies can invest in winning products, eliminate low performers, identify opportunities for new innovations, and optimize for efficiency.
How is SKU Rationalization Typically Performed…and Why is it Insufficient?
For large companies, performing granular analysis at the SKU level would be a seismic task, especially if the analysis is manual. Thousands of SKUs would need to be audited along with the many factors affecting their performance.
In actuality, companies tend to group and analyze SKUs based on a limited number of factors.
One way of measuring SKU performance involves comparing SKUs in the same category. For example, a company might compare the performance of all its sparkling waters against each other, rather than comparing the category to its soda products.
This approach is the most accessible way to analyze SKUs, but it’s lacking because category is a single performance factor among many.
A flavor of sparkling water that’s only sold in one region may perform poorly compared to a national flavor but perform well compared to other regional products.
Grouping SKUs by category doesn’t tell the whole story or show meaningful product performance, which can lead companies to draw the wrong conclusions.
The lack of visibility is a cumbersome hindrance in the way of organizations’ success.
Data science, however, provides a different approach. Data science enables accurate decision-making by analyzing SKUs with the business case in mind.
Machine learning (ML) algorithms can consider every important factor, which isn’t possible for a human analyst contending with deadlines. Additionally, ML speeds up the analysis process significantly, delivering compelling insights directly to business teams within minutes, on-demand.
It can also optimize SKU rationalization on the granular level. Instead of only comparing SKUs within the same category, SKUs can be compared based on likeness. While two different flavors of sparkling water can appear to be similar enough to draw conclusions about performance, this assumption is based on limited characteristics.
ML analyzes many elements beyond just category, allowing the company to gain the greatest amount of insight into SKU performance.
In the next section, let’s further discuss how to use data science and ML to automate SKU rationalization.
A Different Approach: Applying Machine Learning to SKU Rationalization
ML can automate SKU rationalization by analyzing the many factors that affect SKUs. It can then group SKUs together based on overall performance, showing a big picture view of product portfolio health.
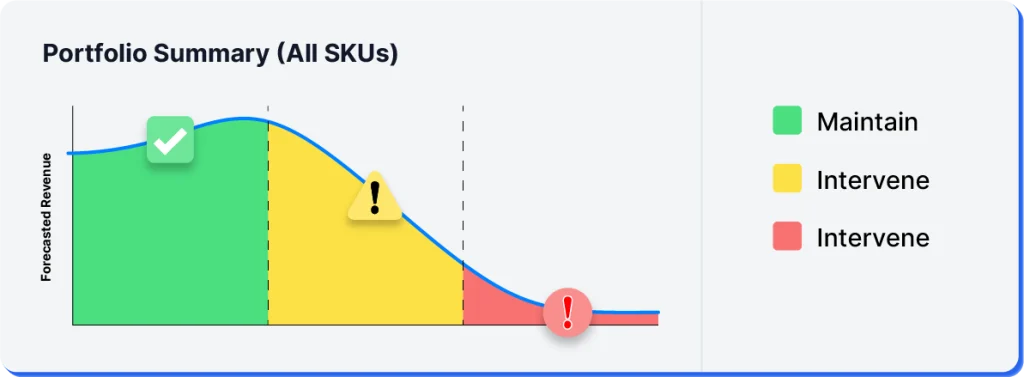
This approach groups SKUs as high, medium, and low performers. This performance is determined by a number of factors, including comparisons with SKUs in and outside the category, as well as competitors, and the overall trend of the segment, retailer, and region.
ML also enables teams to generate granular, SKU-level recommendations. While manual SKU rationalization would require significant translation, ML can automatically serve these recommendations in natural language.
Natural language generation enables a business person to receive recommendations like “cut this item” or “intervene in 2023.” Essentially, business teams can take action without waiting for back-and-forth with an analyst (and the analysts are freed from translating the minutia of their findings).
Recommendations to intervene should be bolstered with forecasts that show future SKU performance by year.
The automated process compresses the workflow and produces compelling, on-demand insights. Instead of yearly, SKU rationalization can occur daily, weekly, quarterly, or monthly to suit the needs of the organization.
The continual analysis is a huge competitive advantage among changing consumer behavior. Companies have instant access to actionable insights from relevant data, rather than data that is weeks or months old.
How, then, do we correctly automate the SKU rationalization process to ensure our companies have access to actionable insights?
We’ll explore the essential elements of automating SKU rationalization and how to successfully incorporate ML software into the process in the next paragraph.
The Essential Elements of Automating SKU Rationalization
The “learning” part of ML starts with successful examples that can be used to teach a machine how to do something.
For a machine to learn SKU rationalization, the first step is to use human insight that identifies high-performing SKUs from which the machine can learn. This is an example of how ML cooperates with human experts to generate successful, scalable results.
ML software creates an idealized model of great SKUs based on examples from human experts. This model is then applied to all SKUs to assess current and probable future performance.
Using historical results, machine learning can create a view of how SKUs will sell over time. This forecasting capability of ML allows organizations to predict SKU performance.
To test whether the forecast is accurate, machine learning models can be used to “predict” what has already happened. This is called backtesting, and it should be used to establish whether ML has accurately learned how SKUs perform.
In SKU rationalization, the goal is to decide how to use limited resources to improve the performance of a large number of SKUs. Price, promotion, distribution, advertising, and innovation all are levers that a marketer can pull to change the trajectory of a SKU.
Deciding which of those levers to use one SKU at a time is ideal, but tedious and expensive, doing so for arbitrary groups is not likely to create optimal results. A better answer is to use ML for this task.
ML is used to make groups out of SKUs according to their characteristics such that marketers can act on these groups instead of on each SKU, while still achieving near-optimal results. The ultimate question is then: “How do we know what actions to take for each group of SKUs?”
The groups of SKUs that ML assembles should make sense to the human experts that provided the training information. If the groupings don’t make sense, the machine learning algorithm likely needs additional iterations with more specifics on which factors are important when determining how to group SKUs.
Once the groups do make sense, the actions needed to be taken will be clear to the marketers receiving the analysis.
Of course, some products will be too new to be meaningfully evaluated. A good ML model should highlight those innovations and exclude them from analysis. Products that are approaching the end of their shelf life should also be excluded.
Apart from the technical essentials, successful SKU rationalization automation requires intelligent change management. Company leaders must endorse AI and ML automation to ensure it’s adopted from a top-down approach. Since this analysis will enable cross-functional collaboration, teams should be aligned from the start of adoption.
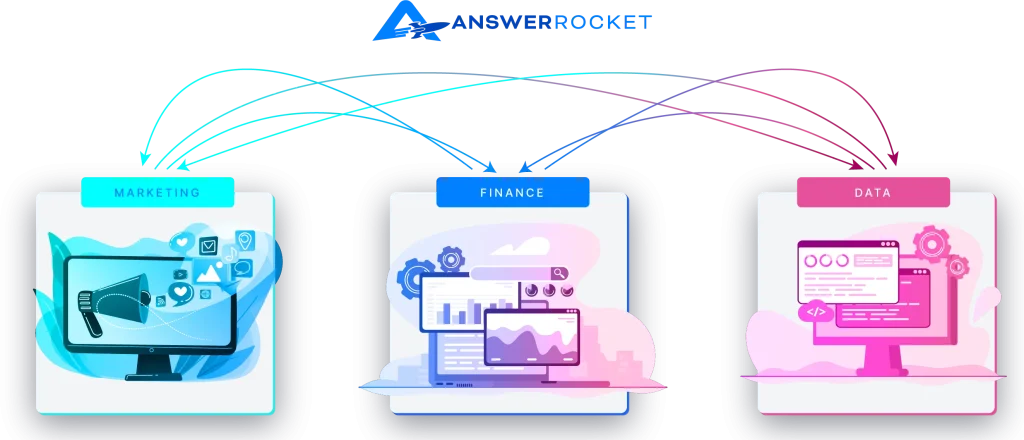
The benefits of this alignment are clear: marketing, finance, and data teams often don’t have enough visibility into each other’s realms. A standardized approach to SKU rationalization creates a clear through-line for decision-making on which products to keep, promote, or cut.
Conclusion
Analytics from automated SKU Rationalization provides companies with the SKU-level insights they need to make better investment decisions and increase the health of their portfolios.
It reduces costs as companies can proactively cut the tail on low-performing SKUs and invest in winners based on predictive insights. They can also track the performance of all SKUs over time.
The real-time analysis provides organizations with a competitive advantage compared to other companies that have not adopted this type of technology. The automation streamlines the entire SKU rationalization process by providing team members with ease of access and more granular insights.
To illustrate, National Beverage, a company with a distinctive portfolio of sparkling waters, juices, and carbonated soft drinks, needed to obtain actionable insights to solve complex problems like SKU rationalization.
The organization utilized AnswerRocket’s AI-powered analytics software to understand what exactly was driving category and product performance with efficient and timely insights, eliminating “analysis paralysis.” Learn more about this use case.
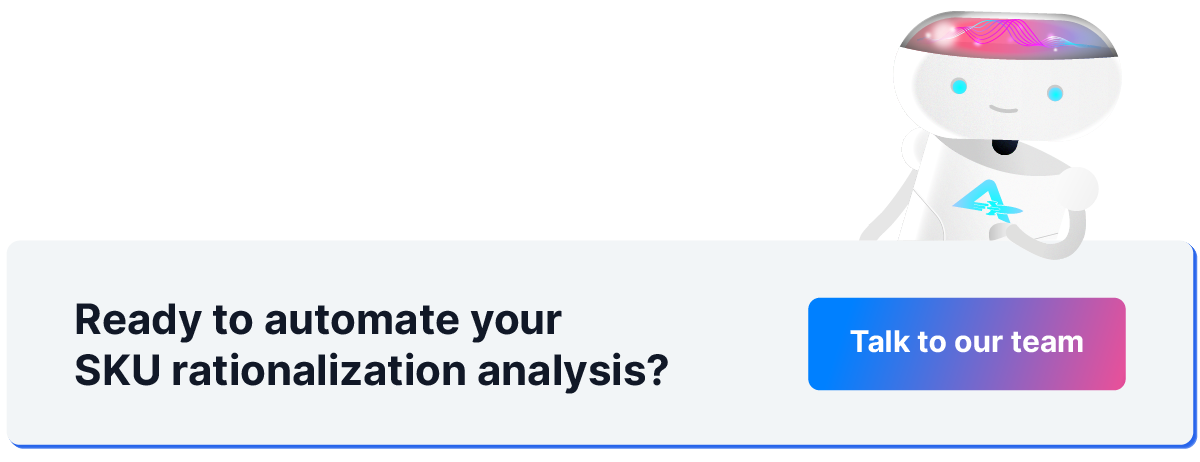
Originally published August 3, 2021.