Benefits of Retail Analytics
The Current State:
Roadblocks to Retail Data Analytics
Unfortunately, it’s not uncommon for retailers to miss out on the potential benefits of analytics for the following reasons:
Historically, retailers have lagged behind CPGs when it comes to investments in analytics.
While retailers are investing more YoY, they don’t necessarily have the budgets to hire more analysts— yet the demand for insights is increasing. As a result, analytics resources are stretched too thin to deliver their full value.
Manual analytics processes are slow and cumbersome.
Currently, analysts must spend time merging and cleaning disparate data sources before manually testing their assumptions against the data. This process is time-consuming and prone to bias. As a result, business people are stuck waiting for answers and analysts spend significant time on routine reports just to understand what happened in terms of performance.
Outdated analytics tools are not sufficient.
Retail analytics solutions traditionally involve business intelligence tools and dashboards. Dashboards focus on illustrating past performance. Though business people need dashboards to make decisions, they can’t easily drill down into data points or answer follow-up questions without technical support. What retailers need are tools that enable better planning and agility via forecasting, trend analysis, and self-service functionality.
While retailers have an abundance of data, their analytics tools and processes are — by and large — not up to par to achieve the real benefits of data analysis. The current state of retail analytics is common, but changing. The digital transformation brought by COVID-19 is one contributor. The other is the advent of more advanced technology, like AI, machine learning, and natural language generation.
Common Pain Points in Handling Consumer Data
Retailers aren’t getting enough value from syndicated data
Retailers know they need syndicated data to be competitive, but many don’t have the tools to generate enough ROI on the investment. How should retailers approach the data? How do they merge panel data with shopper and loyalty data to better understand category and brand performance in context? These questions represent roadblocks for many retailers.
Insights bottlenecks prevent proactive decision making
Retailers often lack real-time insights. In actuality, business teams (like category managers) rely on analysts to produce insights and answer questions. Analysts often get overloaded with ad-hoc requests and routine reporting, meaning analysis can take days or weeks to get out the door. By the time growth opportunities are uncovered, they may no longer be relevant. Retailers have the data they need to gain a competitive advantage, but bottlenecks stifle successful execution.
Data silos block cross-functional collaboration
When marketing, finance, and supply chain are operating against their own sets of data, collaboration between departments is challenging. More importantly, data silos prevent retailers from gaining a holistic picture of business performance. For example, financial analysts may uncover anomalies in revenue without any visibility into the reasons why— unprecedented category growth, out-of-stocks due to pantry-loading, etc. As a result, retailers miss out on growth opportunities, and teams can’t operate as effectively as they could.
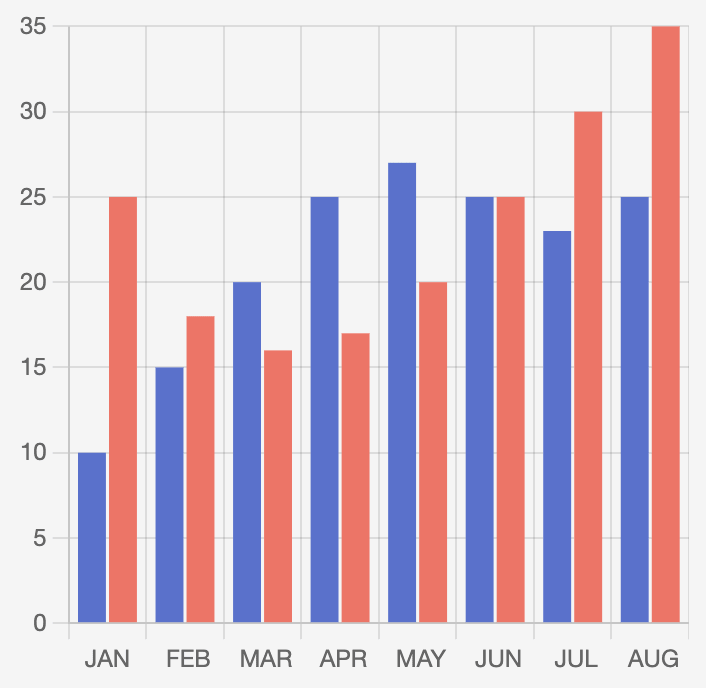
How to Succeed with Retail Data Analytics
1. Centralize data for a single source of truth
Both internal and syndicated data sources are critical for understanding performance in the context of competitors. Retailers need to access shopper, loyalty, and panel data in a single interface to gain the visibility they need to make decisions.
In addition, centralized data enables different teams to collaborate against a single source of truth— meaning, the same question will return the same answer.
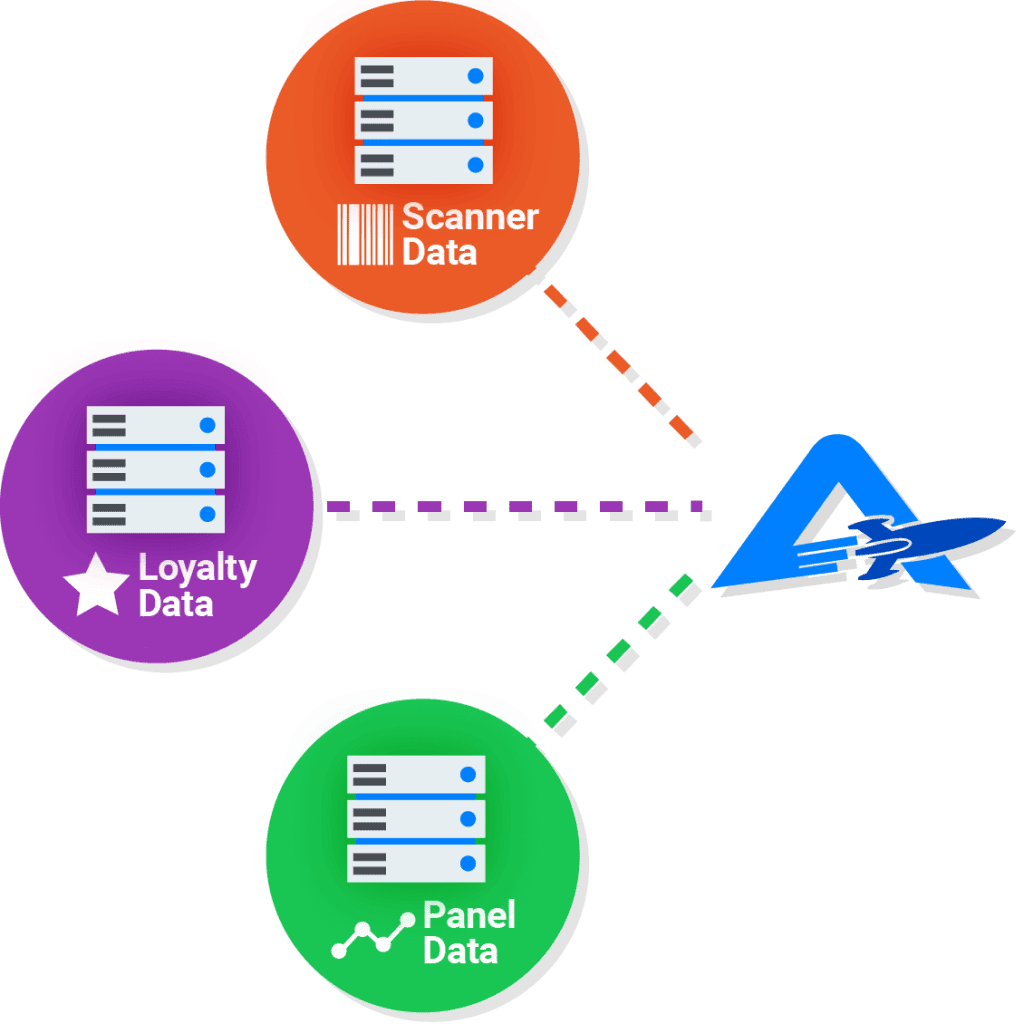
2. Understand performance drivers
Amid volatility and rapidly changing consumer behavior, it’s difficult to determine the root causes behind business performance. For retailers like grocers, pandemic purchasing habits have led to growth as consumer goods fly off the shelves. In contrast, apparel and cosmetics brands are facing steep declines in sales.
On the surface, the reasoning behind these results is intuitive— people were stocking up their pantries due to concerns about the pandemic, and shutdowns have decreased demand for new clothes and cosmetics products as more people live and work from home.
However, simply calculating the numeric changes in performance and stopping with assumptions is not sufficient for long term success. For one, every retailer can make the same assumptions. There’s a competitive advantage to a more granular and precise view of performance drivers.
It’s one thing to know that the rice category grew by 80% last quarter. It’s another thing to know that private label outpaced rice category growth primarily due to promos, but lost $300,000 in potential revenue due to out-of-stocks.
With this knowledge, retailers can make decisions that account for the factors that matter most, investing their resources in the areas that can generate the most ROI.
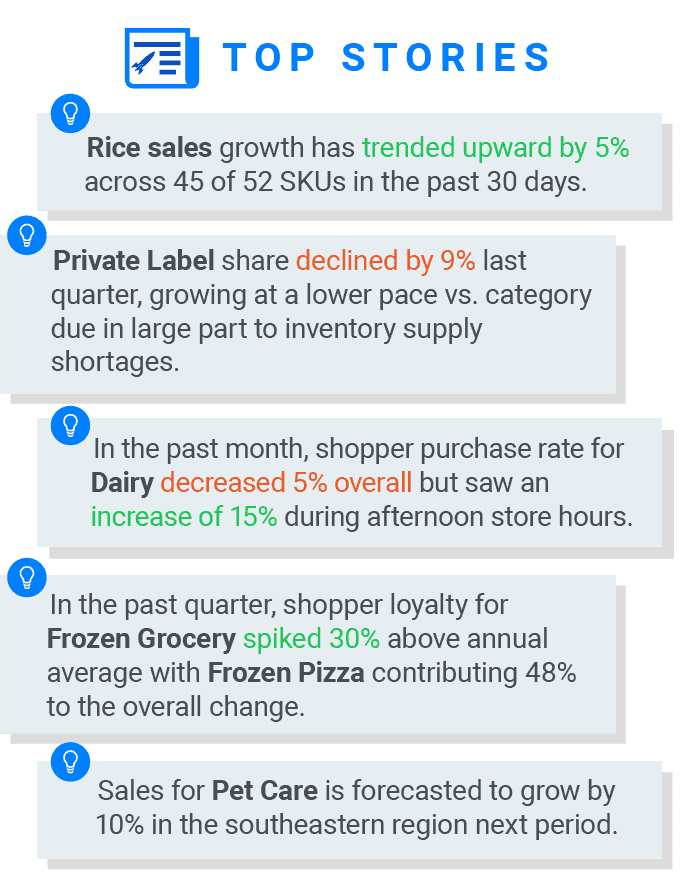
3. Maximize analytics resources
The manual analytics process does not enable retailers to act with speed and precision, especially when business people can’t access data directly. Retailers with small analytics teams are almost definitely feeling the pressures of insights bottlenecks.
So, how can retailers make the most of the analytics resources they do have? Wherever possible, retailers should enable self-service analytics. In practice, this means business people are able to answer their own ad-hoc questions instead of reaching out to an analyst for support. The benefits of self-service analytics are two-fold:
A. Business users can get answers directly, speeding up the turnaround time for insights by orders of magnitude (seconds, compared to days or even weeks)
B. Analysts can dedicate more time to high-value analysis instead of routine reports and ad-hoc questions.
To scale, replicating the best-in-class approaches of their most skilled analysts using AI automation. First, identify the leading analysis use cases to be automated. Analyses that are time-consuming and needed on a routine basis are good candidates. Once the ideal workflow has been identified, retailers should prioritize that workflow for automation, which we’ll discuss more in the next point.
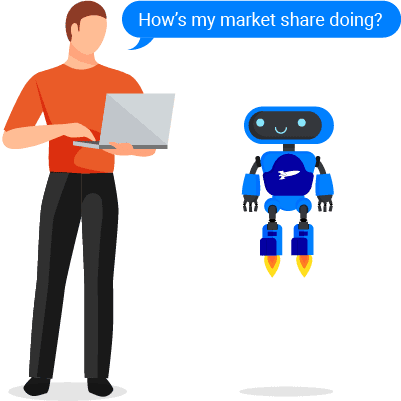
4. Identify opportunities to automate analysis
Automation will be the largest differentiator among retailers in the next decade. What does automation mean today?
Automation is enabled by AI. Specifically, machine learning algorithms centralize data and perform exhaustive analysis. That means these algorithms test every data combination to determine performance drivers, trends, outliers, and more. The algorithms then produce insights in the form of data visualizations and natural language narratives, including charts and graphs accompanied by plain English summaries of what happened and why.
Retailers can begin by automating recurring analytical workflows for their less technical business users. This means that any person on any team could ask a question like “how did the rice category perform last quarter?” and arrive at the same answer— one that’s exhaustively researched and fully contextualized, laying out performance drivers and actionable insights.
Since automation reduces the speed to insight from days to seconds, retailers can act with much more agility and flexibility, making proactive decisions with real-time information.
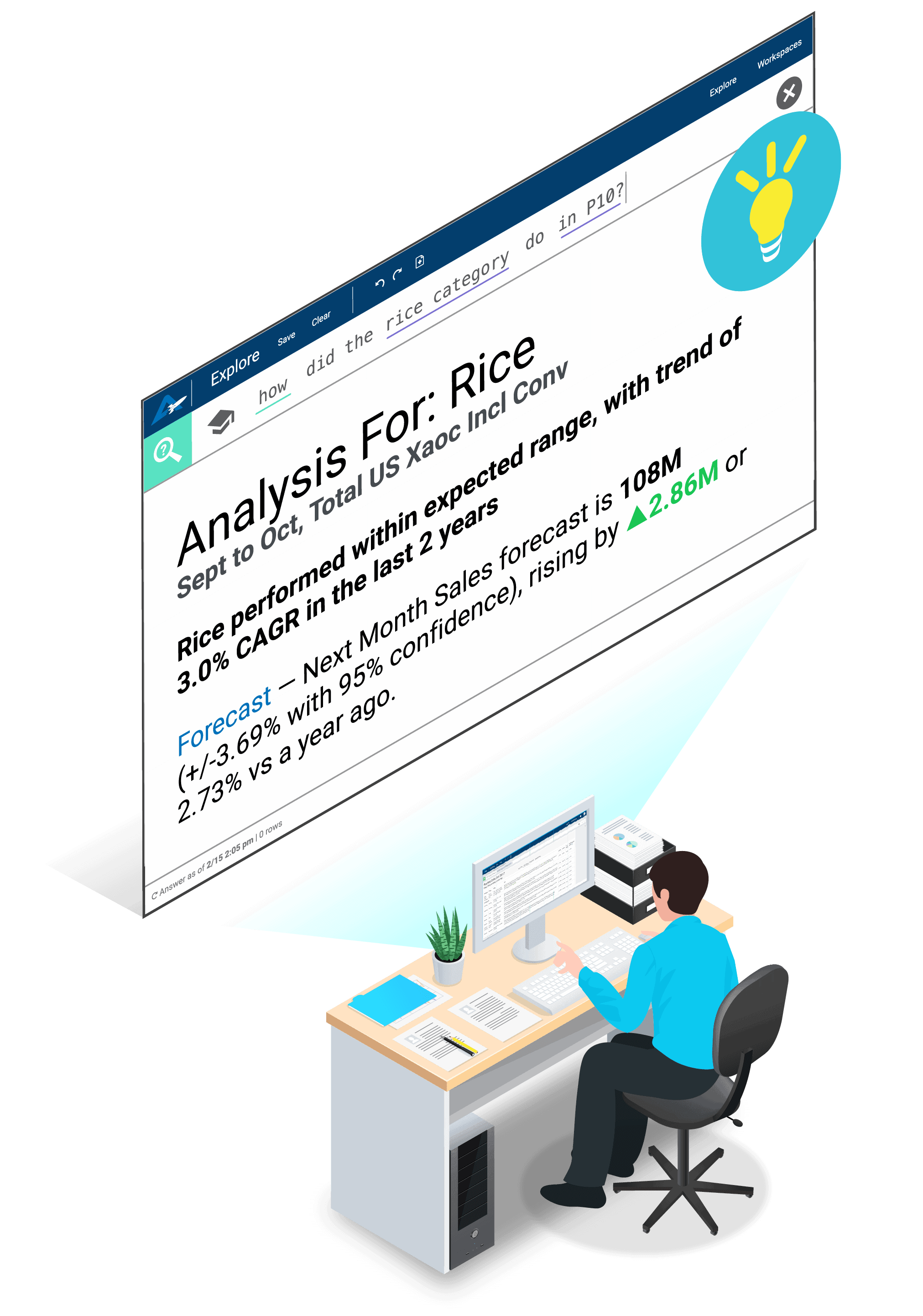
What Can Retailers Automate Today?
Consumer Analysis
Driver Analysis
Market Share Analysis
Demand Forecasting
Choosing the Right Analytics Tools for Retailers
Retailers may be wary of investing in new analytics tools, especially given the uncertainty of today’s economic climate. However, analytics tools that enable better decision-making drive top line growth. When hiring new analysts isn’t viable, when syndicated data is a costly investment, and when retailers need certainty amid an uncertain time, the right analytics solution can make the difference.
Manual analytics processes and static dashboards won’t cut it— retailers should consider a new approach.
A New Approach: Augmented Analytics for Retailers
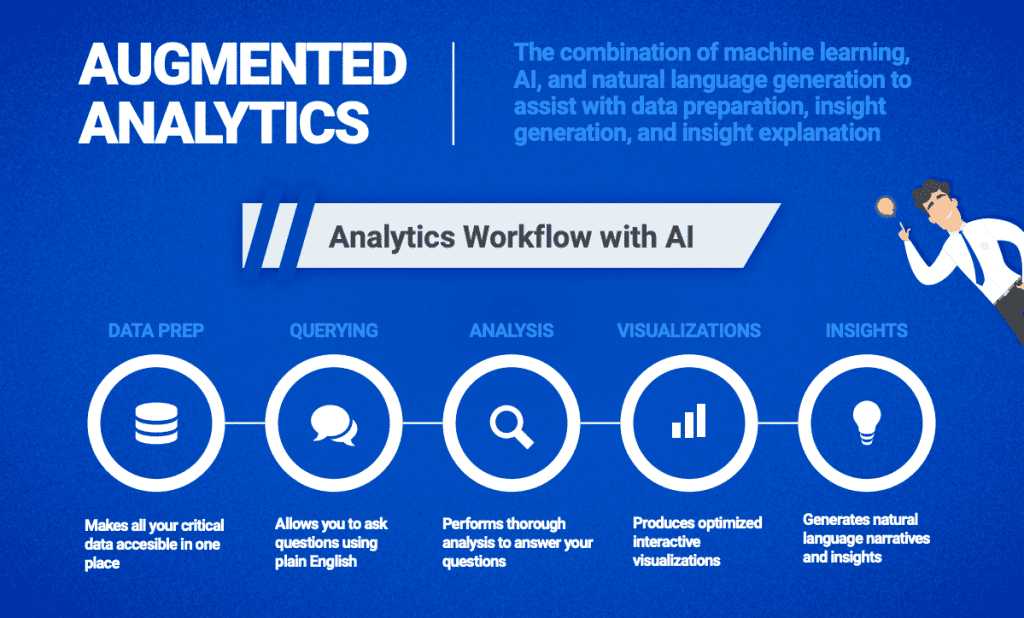
Core Functions for Successful Retail Analytics
AnswerRocket is an advanced analyticEnabling business users to answer their own ad-hoc questions and generate routine reports requires the right functionality— features that speak to the business user experience rather than just the analyst.