As consumer data grows, so too do the opportunities to better understand and target customers and prospects.
To capitalize on this data, businesses must frame their approach strategically. After all, having the data is not enough to:
- Interpret and understand the story it’s telling.
- Determine which data is most relevant to which audience.
- Instill a culture of data discovery in employees, especially when acting on hunches can be habitual.
Business leaders understand the value of data that’s tailored to each function and the role analytics tools play in the overall employee experience of accessing that data.
In this sense, analytics software that organically promotes data-driven decision-making provides a competitive advantage.
The advent of AI analytics has changed the premise of the conversation. With the automation and augmentation capabilities of AI, analytics tools are no longer facilitators of data analysis but are capable of performing the actual labor that was once unique to humans.
These advancements mean that businesses have an incredible opportunity to capitalize on data (as we’ve mentioned), but they must do so with an eye toward scale, change management, and curiosity culture.
In this article, we’ll specifically discuss the advantages of machine learning analytics and how it fits into the larger picture of AI in business intelligence.×
The difference between traditional data analytics and machine learning analytics
Data analytics is not a new development. From the beginning of business intelligence (BI), analytics has been a key aspect of the tools employees use to better understand and interact with their data.
However, the scale and scope of analytics has drastically evolved. Let’s discuss these differences in more detail.
Data Analytics
Traditional data analytics platforms typically revolve around dashboards.
Dashboards are constructed of visualizations and pivot tables that illustrate trends, outliers, and pareto, for example. Technical team members like data analysts and data scientists play a role in constructing these dashboards; generally, the humans are still performing the bulk of the analysis, and the software helps facilitate the results.
Current state analysis with traditional data analytics software looks something like this:
- The data analyst starts with a core question, likely sourced from a business team. In this case, the question is “how did market share do last quarter?”
- The data analyst accesses different spreadsheets from different locations.
- The data analyst merges multiple spreadsheets manually.
- The data analyst conducts analysis by filtering data based on their hypotheses around market share’s performance. This process is constrained by time restrictions, so the analyst can’t fully test every scenario. As the analyst iterates on their hypotheses, they may need to access data again.
- The analyst presents the story, or the findings from their analyses. While these stories can be well-researched and accurate, they’re not a complete picture of what’s happening in the data and rely on the analyst’s initial assumptions.
This process is labor-intensive, time-consuming, and often frustrating. Data analysts have advanced skill sets that they can’t use effectively when they’re spending their time stuck in a cycle of routine reports.
The limitations of this process have paved the way for machine learning to take hold in analytics.
Machine Learning Analytics
Machine learning analytics is an entirely different process.
Machine learning automates the entire data analysis workflow to provide deeper, faster, and more comprehensive insights.
How does this work?
Machine learning is a subset of AI that leverages algorithms to analyze vast amounts of data.
These algorithms operate without human bias or time constraints, computing every data combination to understand the data holistically. Further, machine learning analytics understands boundaries of important information.
If asked to identify changes in sales figures, the machine can learn the difference between a $200 fluctuation and a $200,000 increase, only reporting the latter because that’s the info that actually impacts the company.
In other words, machine learning also tests out hypotheses to answer key business questions — but it can test all of them in a much shorter timespan. Think seconds instead of weeks. Then, it tells a data story that’s accurate, exhaustive, and relevant to the person asking questions.
Practically, machine learning is invoked in techniques like:
- Clustering — The machine determines commonalities between different data to understand how certain things, like customers, are alike. These customers can be grouped together in ways that may not be immediately apparent or intuitive to a person performing the same exercise.
- Elasticity — The machine determines causes behind results. If many factors are changing simultaneously, how do you determine which factor is credited with which outcome? This technique tells employees that an increase in household income resulted in boosted sales, rather than product promotions, for example.
- Natural language — The machine maps phrases like “sales” to their coding language counterparts. In this way, business people don’t have to understand R or Python to perform deep analysis. They can simply ask everyday questions like “what were sales in Q2?” and the machine translates those words.
With these techniques, machine learning analytics determines the drivers beneath the data and the opportunities to grow the most.
Significantly, machine learning that invokes natural language is also targeted toward business users who can perform the analysis themselves (a development known as augmented analytics).
Machine learning analytics are taking off…but why now?
The amount of data that companies have access to is much greater now than it has ever been before.
This data is a goldmine for businesses as it can inform the decision-making process, assist with targeting customers and prospects, and deepen the level of analysis that can be performed.
However, as the amount of data grows, so too do the challenges with harnessing its power:
- The roles and functions that make data-driven decisions are often removed from the data itself. CMOs, brand managers, sales teams, and other business-driven roles need data to act, but don’t have the time or training to divulge insights from the data without user-friendly tools or support from technical team members like data scientists and analysts.
- The data itself is more complex. Businesses need to invest resources into data cleaning, structuring, and maintenance to ensure that data pipelines are supported properly.
- The value of data is becoming more apparent. As more businesses invest in syndicated data sources, how do businesses gain a competitive advantage, especially when competitors are accessing the same data?
In tandem with this growth in data is a growth in computational processing power.
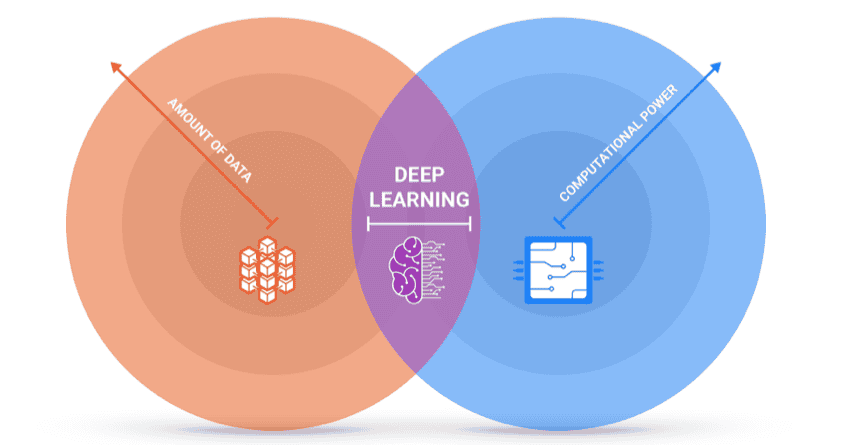
Cloud computing, the technology that ultimately supports this data, is becoming more advanced, and machines have more processing power than they have previously.
Companies are investing in both big data and cloud infrastructure.
According to SVP Pete Reilly in this CGT webinar, they’re investing toward an AI-driven end:
“They’ve got all this data available, and now they’re saying, what are the big business problems we could apply this to that would have a huge impact?”
After all, at the intersection between the expansion of data and computational power is machine learning.
Machine learning is essentially what you do with these resources to leverage them as business assets. Without machine learning, companies simply have a sea of disparate information. With machine learning, companies have a hierarchical structure of the information that’s most specific, relevant, and important to each role and function.
As indicated in Reilly’s quote, specific business problems can focus the implementation of machine learning.
Key considerations for data analytics and machine learning
The potential gains from machine learning have enormous appeal, and companies are looking to invest in advanced analytics solutions.
Yet — as with the larger conversation around AI in business — the pathway to successful implementation of machine learning is not as easy as it may appear. Change management strategies are critical for ensuring that employees use machine learning analytics effectively.
Machine learning is new in most industries, and its benefits aren’t necessarily obvious to employees who haven’t been exposed to the larger conversation. This is especially true when employees are concerned about being replaced by automation.
A good implementation strategy is key.
This strategy should be driven by:
- Specific business outcomes that clarify what machine learning analytics will accomplish and automate.
- Alignment between tech and business teams, so that both parties understand the benefits of workforce augmentation.
- Accurate data, supported by system maintenance and AI expertise.
- Change management fundamentals, which are often lost in the excitement of new technology.
These considerations will help ensure that machine learning analytics take root in the business and help employees become more effective in their jobs.
Request a Demo
See how AnswerRocket leverages machine learning to transform data analytics.